Table of Contents
Introduction
Data warehouses and data lakes are both rapidly used to store large amounts of data for analytics, but these two are not interchangeable terms. A data lake is a massive repository that tends to include a huge amount of raw data, but the specific purpose for this has not been defined. However, a data warehouse is a powerful repository for filtered, structured data that has already been processed for a defined purpose.
The distinction is essential because they both have different granular purposes and need different sets of eyes to be optimized properly. Understanding this contrast empowers businesses to architect a data infrastructure that optimally balances structured efficiency with unstructured potential, thereby driving strategic decision-making, analytics agility, and future growth. This article delves into the intricacies of these two concepts, offering insights into when and how each should be employed to extract maximum value from data resources.
Importance of data warehouse and data lake for your organization:
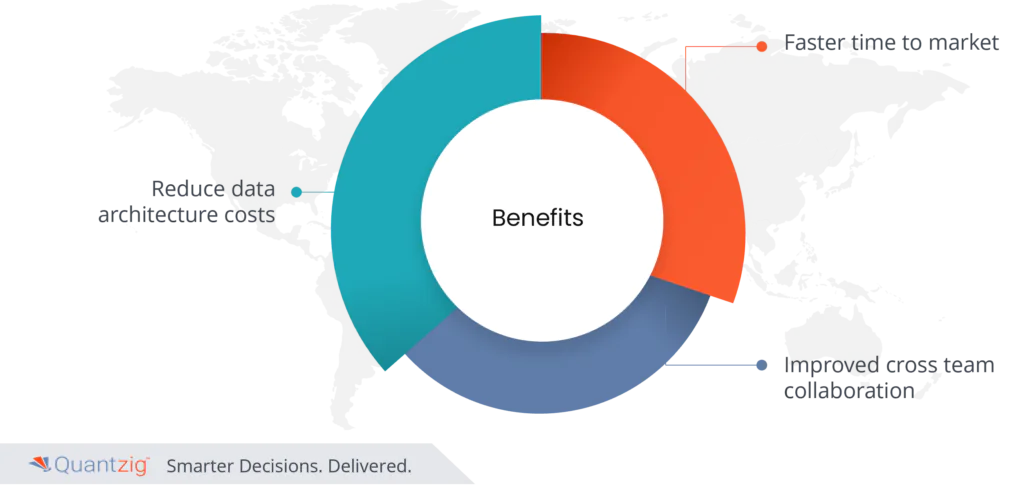
- Reduce data architecture costs:
If you gain a comprehensive understanding of the distinctions between data lake and data warehouse, you can get substantial cost savings within data architecture. By correctly identifying the use cases for each platform, businesses can allocate resources more efficiently. Data warehouses are ideal for structured data requiring high-speed queries that make them cost-effective for critical business analytics. On the other hand, data lakes accommodate unprocessed, raw data at a lower cost which makes them suitable for storing a huge amount of unstructured data for future analysis. As a result, by avoiding the misallocation of data and utilizing the strengths of each platform, redundant infrastructure expenses can be reduced. Besides, the users can prevent unnecessary investments in incompatible tools after selecting suitable storage technologies and processing frameworks.
- Faster time to market:
Data warehouses excel in delivering rapid insights from structured data that enable quicker response to market trends and customer demands. Their optimized schema design and indexing facilitate swift querying, aiding in timely decision-making. Simultaneously, data lakes offer a haven for raw and unstructured data. Their flexibility allows businesses to swiftly onboard new data sources without prior structuring, which is particularly advantageous in rapidly evolving markets. This agility can hasten the experimentation and innovation processes and enable organizations to swiftly test new ideas and iterate products faster.
- Improved cross-team collaboration:
A broad knowledge of the difference between a data warehouse and a data lake enables diverse teams, such as engineers, data analysts, and business stakeholders, to work cohesively to foster a more holistic approach to data utilization. We know data warehouses provide a structured environment for analytics, so, it is easier for teams to collaborate on standardized data sets. Consistent data models and query languages streamline communication, allowing teams to share insights seamlessly. On the other hand, data lakes offer a flexible repository for diverse data types. This promotes collaboration by accommodating various data sources without the need for immediate structuring, fostering a more inclusive approach to data exploration. Cross-functional teams can collectively access and analyze data, that contribute to innovative business ideas and collaborative problem-solving.
Conclusion:
The conversation between “data lake vs data warehouse” has likely just begun, but the main differences in process, users, structure, and overall agility make both models unique. Based on your organization’s unique requirements, developing an accurate data warehouse and data lake will be instrumental in long-term growth. Primarily, many organizations use data warehouses, and the objective is toward cloud data warehouses. On the other hand, data scientists typically use Data lakes for the exploration of flat files and machine learning. Still, many organizations use both two platforms to fulfill the spectrum of their data storage requirements.
Success Story:
Data Synergy Unleashed: How Quantzig Transformed a Business with Successful Integration of Data Warehouse and Data Lake
Client Details: A global leading IT company
Challenges:
- Fragmented and duplicated solutions:
For the client, dealing with fragmented and duplicated solutions posed significant challenges in their operational landscape. Multiple teams within the company had independently developed solutions to address specific tasks or departments which led to redundancy, inefficiency, and inconsistent data practices. Fragmentation hindered effective decision-making as crucial insights were scattered across various systems. On the other hand, duplicated efforts led to confusion, as different teams used disparate tools to achieve similar goals. Maintaining and updating multiple solutions consumed unnecessary resources and drained both time and money.
- Separate data pipelines for each project leading to high utilization of computing resources:
The client was maintaining separate data pipelines for each project which resulted in excessive utilization of computing resources. With multiple projects running concurrently, each with its own dedicated pipeline, computing infrastructure was under strain due to inefficiencies and overallocation. This approach led to resource wastage as projects with varying resource requirements couldn’t dynamically share or allocate computing power. This resulted in underutilization in some pipelines and contention for resources in others led to longer processing times, increased costs, and operational bottlenecks. Moreover, managing numerous pipelines became complex, as it required additional administrative efforts and made scalability difficult. As a result, the client couldn’t respond quickly to changing demands or scale resources as needed for specific projects.
- High manual maintenance of data pipelines:
The company relied extensively on manual maintenance of data pipelines which resulted in increased human errors, slower response times, and a drain on human resources. Frequent maintenance requirements such as data format changes or pipeline adjustments consumed valuable time that could have been directed toward more strategic tasks. During manual interventions, the risk of errors also posed data quality and integrity concerns and led to incorrect insights or decisions. As the company expanded and data volume grew, the existing manual maintenance approach became unsustainable.
- Recurring service time-outs
The client was facing hurdles with recurring service timeouts, which significantly impacted their operations and user experience. These time-outs occurred when certain services or processes took longer to respond than the established threshold, leading to disrupted workflows and frustrated users. These interruptions impeded data flow and caused delays in critical operations and data processing. As a result, important tasks were left unfinished, and the client’s reputation suffered as users experienced slow or unresponsive services. Frequent timeouts indicated potential inefficiencies or bottlenecks within the infrastructure, which required investigation and optimization efforts.
Solutions:
- Implemented data lake house combining benefits of warehouse and data lake:
At first, we implemented a data lakehouse, which combines the advantages of a data warehouse and data lake, that enabled the client to store, and process structured and unstructured data in a unified environment. By integrating the strengths of both concepts, the company achieved improved data accessibility and ensured various teams could access and analyze data without data silos or duplicated efforts. The ability to perform complex analytics on diverse data types led to deeper insights and informed decision-making. Additionally, Data Lakehouse reduced the overhead of maintaining separate solutions and facilitated automated data processing pipelines.
- Self-healing governance systems to auto-correct data quality and schematic issues:
Our self-healing governance systems automated the correction of data quality and schematic issues. This technology enabled the client to maintain a consistent and accurate data environment without requiring manual intervention. By automatically identifying and rectifying data quality discrepancies and schema inconsistencies, the self-healing system ensured that accurate information was readily available for analysis and decision-making. This led to improved trust in data-driven insights and reduced the risks of erroneous conclusions. The system’s ability to identify anomalies and automatically apply corrective measures saved significant time and resources that would have otherwise been spent on manual data cleansing and validation. This translated into enhanced operational efficiency and faster data processing cycles.
- Data mesh architecture for domain and local teams to develop on top of centralized architecture:
The adoption of a data mesh architecture empowered the client by facilitating domain and local teams to build on a centralized architecture. This approach decentralized data ownership, and enabled teams to take charge of their data domains while adhering to standardized practices. With a domain-oriented team responsible for data quality, modeling, and operations, the client achieved improved data accuracy and relevance. Local teams were empowered to develop solutions tailored to their specific needs, fostering innovation and agility in analytics and insights. While the centralized architecture provided a foundation of shared services (such as data storage, processing, and governance tools), data mesh architecture encouraged collaboration between teams.
- Data marketplace for users to access insight-rich data without knowledge of coding:
Our team implemented a data marketplace that allowed the client to provide users with easy access to data insights without requiring coding knowledge. This user-friendly platform enabled self-service data discovery and retrieval, promoted data democratization and reduced dependency on IT teams. Users could effortlessly search and access pre-analyzed and curated datasets from various sources within the organization. This accessibility to insight-rich data empowered business users to make informed decisions promptly, without the need for coding skills. The marketplace’s intuitive interface and self-service capabilities also expedited data-driven decision-making processes. It reduced the burden on IT teams, freeing them from constant data retrieval requests.
Impact Delivered:
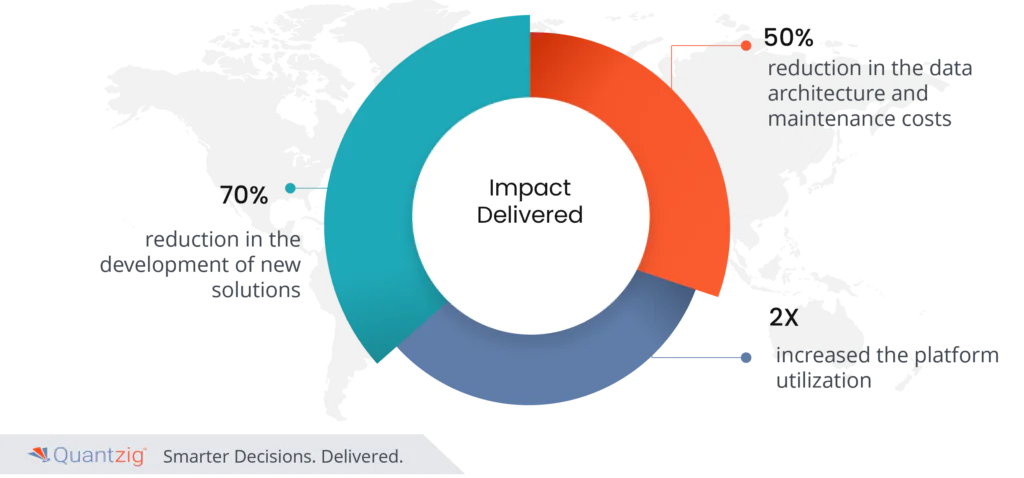
- 70% reduction in the development of new solutions
- Reduced the data architecture and maintenance costs by 50%
- Increased the platform utilization by 2X.
Want to boost your data landscape? Don’t miss out. Contact Quantzig to explore the limitless possibilities of unified data solutions!