Introduction
In the dynamic realm of the IT industry, staying abreast of evolving trends is pivotal for organizational growth and success. Data analytics has emerged as a transformative force, enabling IT businesses to harness the power of information for enhanced performance and strategic decision-making. Predicting Trends in the IT Industry is a crucial task that helps businesses stay ahead of the competition and make informed decisions. By leveraging advanced technologies such as artificial intelligence (AI) and machine learning (ML), organizations can gain valuable insights from large amounts of data.
In this analysis, we will explore the implementation, design, and testing phases of product containers, as well as their significance in the financial industry. Additionally, we will discuss the role of AWS in managing data domains and the importance of data lakes and enterprise data catalogs in organizing and accessing high-quality data. Furthermore, we will shed light into the concept of synthetic data and its applications in training AI/ML analytics systems. Let’s explore the current trends shaping the IT industry through the lens of analytic tools.
Analytics in the IT Industry
These techniques are an assortment of business intelligence, online analytical processing (OLAP), and other advanced applications with a much broader focus. These services have expansive organizational benefits that help businesses drive revenue, improve operational efficiency, optimize customer experience, and develop effective marketing campaigns. Data analytics coupled with business intelligence and related advanced analytical services can work wonders in every industry, especially in the booming IT industry.
With growing competition and the presence of a highly fragmented market, it is essential for the players in the IT sector to respond quickly to the latest market trends to gain a competitive advantage over their competitors.
The IT industry is witnessing a significant shift towards the adoption of data product containers. These containers provide a standardized and scalable environment for implementing, designing, and testing data-driven solutions. By encapsulating data and its associated dependencies, organizations can ensure consistent and reproducible results across different computing environments. For example, in the financial industry, JPMorgan Chase Bank has embraced data product containers to streamline their analytical workflows and enhance collaboration among scientists and engineers.
Another emerging trend is the utilization of AWS services for managing domains. AWS offers a comprehensive suite of tools and services that enable organizations to store, process, and analyze vast amounts of data securely and efficiently. By leveraging AWS’s scalable infrastructure, businesses can harness the power of cloud computing to derive actionable insights.
Data lakes and enterprise catalogs are becoming indispensable components of modern analytics architectures. These tools provide a centralized repository for storing raw and unstructured data, enabling organizations to perform advanced analysis and derive valuable insights. On the other hand, enterprise catalogs facilitate the discovery and understanding of available assets, ensuring governance and compliance.
The concept of synthetic data is gaining traction in the IT industry, particularly in the development and training of AI/ML analytics systems. It refers to artificially generated data that mimics real-world characteristics. It offers several advantages, including privacy preservation, data augmentation, and the ability to create diverse and representative datasets. ML models trained on fully or partially synthetic data can yield accurate predictions while protecting sensitive information.
Book a demo to experience the meaningful insights we derive from data through our analytical tools and platform capabilities. Schedule a demo today!
Request a free demo
What’s Trending in the IT Industry?
The ultimate goal of any business, irrespective of the industry it operates in, is to boost business performance and drive profitability. The IT industry possesses large amounts of information – historical and real-time – from a variety of sources that can be processed and analyzed with the help of analytical tools. With the help of analytical tools, the IT industry players can leverage this data to reduce costs, optimize processes, and enhance organizational operability. But what are the trends that will revamp the IT industry? Read on.
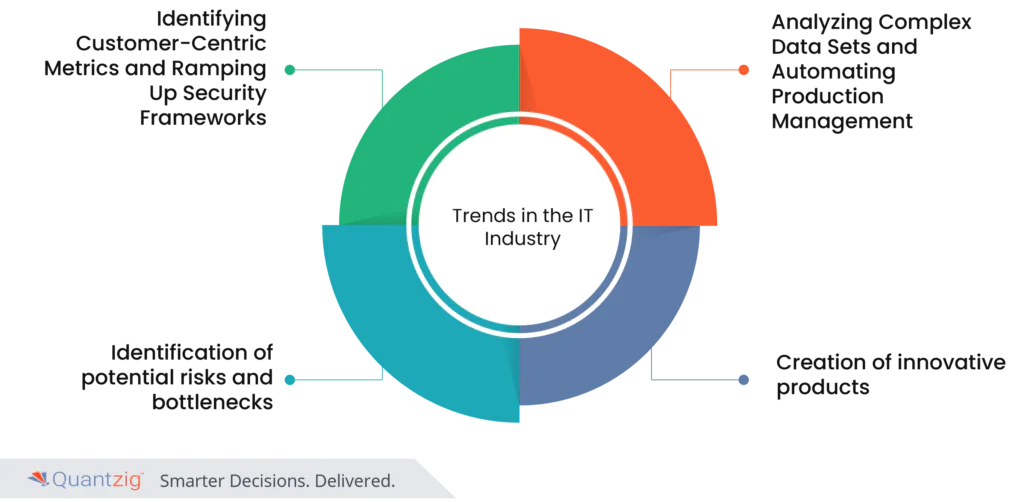
1. Identifying Customer-Centric Metrics:
- Enhanced Customer Experience:
The IT industry is increasingly leveraging this technology to identify customer-centric metrics, measuring and monitoring performance across various parameters. This enables businesses to track brand perception, gauge customer satisfaction, and tailor services for an enhanced customer experience.
2. Ramping Up Security Frameworks:
- Data Security Prioritization:
With the rise of technology, there’s a concerted effort in the IT industry to bolster security frameworks. This proactive approach aims to eliminate security issues, safeguard sensitive information, and fortify systems against vulnerabilities, ensuring integrity and user trust.
3. Analyzing Complex Data Sets:
- Seamless Business Processes:
IT vendors are increasingly relying on advanced software to analyze and interpret complex data sets. This trend simplifies business processes, ensuring operational efficiency, a seamless experience, and extracting valuable insights for informed decision-making.
Experience the advantages firsthand by testing a customized complimentary pilot designed to address your specific requirements. Pilot studies are non-committal in nature.
Request a free trial now
4. Automating Production Management:
- Reducing Human Intervention:
This technology is enabling IT companies to automate production management, minimizing the need for extensive human intervention. By analyzing historical data and real-time information, businesses can automate physical changes to equipment, optimizing manufacturing processes through targeted commands to actuators and advanced robots.
5. Identification of potential risks and bottlenecks
- Optimal Risk Management:
Data analytics in IT plays a crucial role in identifying potential risks and bottlenecks. In the ever-changing landscape of technology, having robust risk management processes is essential. It assists in developing optimal risk management solutions and devising agile strategies to navigate uncertainties.
6. Creation of innovative products
- Customer-Centric Innovation:
This platform empowers IT companies to innovate products by collecting and analyzing extensive data on existing market offerings. Insights from customer feedback, product success metrics, and competitor inputs guide businesses in understanding customer needs, driving innovation, and staying ahead in the competitive landscape.
Get started with your complimentary trial today and delve into our platform without any obligations. Explore our wide range of customized, consumption-driven analytical solutions services built across the analytical maturity levels.
Start your free trial nowPredicting Trends in the IT Industry
Cryptocurrency Trends: Cryptocurrencies have gained significant attention in recent years, and their impact on analytic services cannot be ignored. As more businesses adopt cryptocurrencies as a form of payment, there is a growing need to analyze the data generated from these transactions. Predictive analytic solutions can help identify patterns and trends in cryptocurrency usage, enabling businesses to make more informed decisions regarding investments, customer behavior, and market trends.
Data Science Trends: It plays a pivotal role in driving IT trends. With the increasing volume and complexity of data, businesses are relying on scientists to extract valuable insights. Predictive models developed by scientists can help identify patterns, forecast future trends, and optimize business processes. Techniques such as machine learning (ML) and natural language processing (NLP) are used to analyze unstructured data, enabling businesses to gain a deeper understanding of customer preferences, sentiment analysis, and market trends.
AI and ML: Artificial intelligence (AI) and machine learning (ML) are revolutionizing analytic services in the IT industry. AI-powered algorithms can process vast amounts of data and identify patterns that humans may overlook. ML models can predict customer behavior, optimize inventory management, and enhance marketing and sales strategies. Additionally, AI can automate information processing tasks, saving time and resources for businesses. As AI and ML technologies continue to advance, we can expect more sophisticated predictive models that provide accurate insights for decision-making.
Business Intelligence (BI): BI tools and technologies are essential for analyzing and visualizing data in the IT industry. These tools enable businesses to gather, store, and analyze data from various sources, providing valuable insights into key performance indicators (KPIs) and business processes. Predictive models in BI can help businesses identify trends, forecast future outcomes, and make informed, valuable decisions. By leveraging BI tools, businesses can gain a competitive edge by understanding customer behavior, optimizing operations, and improving overall performance.
Edge Computing and IoT: The proliferation of Internet of Things (IoT) devices generates vast amounts of data that can be leveraged for predictive models. Edge computing brings data processing closer to the source, reducing latency and enabling real-time insights. By analyzing data at the edge, businesses can make faster decisions, optimize processes, and improve customer experiences. Predictive models in edge computing and IoT can help businesses detect anomalies, predict equipment failures, and optimize resource allocation.
Data Mesh Architecture: It is an emerging trend that focuses on decentralizing ownership and governance. It promotes the idea of domain-oriented, self-serve platforms that enable teams to manage their data independently. Predictive models in a data mesh architecture allows teams to leverage their domain-specific data to gain insights and make informed decisions. By democratizing data access and analysis, businesses can foster an insight-driven culture and accelerate innovation.
Conclusion
In conclusion, the convergence of analytics and the IT industry is reshaping business strategies, enhancing security measures, and fostering innovation. As businesses continue to prioritize customer-centricity, automation, and risk management, the utilization of data analytics in IT becomes not just a trend but a fundamental driver of success. Embracing these trends positions organizations to navigate the evolving IT landscape with agility, competitiveness, and a commitment to continuous innovation.
Predicting trends in the IT industry requires a comprehensive understanding of various factors such as cryptocurrency trends, data science advancements, AI and ML technologies, business intelligence tools, edge computing, IoT, and data mesh architecture. By staying updated with these trends, businesses can harness the power of analytical services to drive growth, optimize operations, and gain a competitive advantage in the ever-evolving IT landscape. This technology is the one-stop solution to all IT business challenges. The implementation of data product containers, the utilization of AWS services for managing data domains, and the adoption of data lakes and enterprise data catalogs are key trends shaping the industry.
Additionally, the use of synthetic data in training AI/ML analytics systems offers new possibilities for accurate predictions while addressing privacy concerns. By staying informed and embracing these trends, businesses can unlock the full potential of their data and gain a competitive edge in the IT industry. To learn how you can leverage analytical tools to optimize your organization and drive innovation, growth, and market excellence,