Unlocking the Future of Healthcare: Why Predictive Analytics is the Future of Personalized Healthcare
In the healthcare industry, the explosion of data and the rise of innovative technologies have unlocked new ways to improve patient outcomes and streamline operations. At the forefront of this transformation is healthcare predictive analytics, a game-changing approach that utilizes vast amounts of healthcare data to forecast outcomes, optimize patient care, and drive more informed decision-making. As healthcare systems worldwide move toward personalized care, predictive analytics in the healthcare industry is emerging as the key to unlocking the future of personalized healthcare.
Gain limited-time complimentary access to our analytics platform and analyze the true potential of predictive analytics in healthcare decision-making.
Table of Contents
What is Predictive Analytics in Healthcare?
Predictive analytics in healthcare leverages historical and real-time data to create predictive models that forecast future events, from disease progression to patient response to treatments. These predictive models in healthcare are built using advanced algorithms and healthcare analytics software that identify patterns in vast datasets. By analyzing patient histories, treatment effectiveness, and population health data, predictive healthcare tools can provide insights that help healthcare providers deliver personalized, proactive care.
The Benefits of Predictive Analytics for Personalized Healthcare
- Improving Patient Care with Predictive Analytics: One of the most significant benefits of predictive analytics in healthcare is its ability to improve patient care. By identifying high-risk patients and predicting potential health issues, healthcare providers can intervene early and prevent the progression of diseases. This proactive approach is particularly valuable for chronic disease management, where timely interventions can lead to better outcomes and reduced hospital admissions.
- Predictive Analytics for Chronic Disease Management: Predictive analytics for healthcare providers offers tailored solutions for managing chronic diseases such as diabetes, heart disease, and COPD. By analyzing patient data, predictive models in healthcare can help predict disease flare-ups and recommend personalized treatment plans, ensuring that patients receive the right care at the right time. This not only improves patient outcomes but also reduces the strain on healthcare systems.
- Predictive Analytics for Patient Outcomes: Healthcare predictive analytics plays a crucial role in enhancing patient outcomes. By analyzing real-time data from wearables, electronic health records (EHRs), and diagnostic tools, healthcare providers can monitor patient health more closely and make more accurate predictions about patient recovery. This enables more personalized care, where treatments are adjusted based on predicted outcomes, leading to better results for patients.
- Predictive Healthcare Analytics for Hospitals: Hospitals and healthcare facilities can also benefit from predictive healthcare analytics by optimizing resource allocation and reducing operational inefficiencies. Predictive models help in forecasting patient inflow, predicting bed availability, and improving staff scheduling, all of which enhance hospital efficiency and patient care.
Importance of Predictive Analytics in Healthcare
To analyze the benefits of predictive analytics in healthcare, it is imperative for healthcare service providers to acknowledge the myriad ways through which they can benefit from this discipline. Having said that it’s crucial to note that predictive analytics in healthcare plays a crucial role in improving operational management including the overall improvement of business operations, personalization of medicine or drug therapies that assist and enhance the accuracy of diagnosis, and cohort treatment and epidemiology to assess potential risk factors for public health.
Predictive Analytics in Healthcare: What are the risks involved?
Predictive analytics in healthcare holds tremendous potential for transforming patient care, streamlining operations, and improving overall outcomes. However, like any powerful tool, it comes with inherent risks and challenges that need careful consideration. Here, we delve into the key risks associated with predictive analytics in healthcare:
- Technological Advancements: Tech innovations enhance healthcare decision-making but require balancing new tools with traditional practices. Rapid changes can challenge system adaptation.
- Ethical Concerns: While machines offer accuracy, human judgment remains crucial in healthcare. A balance between automation and human input ensures ethical decisions.
- Algorithm Bias: Algorithmic bias can skew predictive analytics. Strong regulations are needed to ensure fair and accurate representation in healthcare data.
Use Cases of Predictive Analytics in Healthcare
Predictive analytics in healthcare offers immense potential to revolutionize patient care, but it is essential to address the associated risks and challenges. From navigating technological advancements and ethical considerations to mitigating algorithmic biases, a holistic approach is required. Here are few of the use cases that proves the requirement of Predictive analysis and how it is a stepping stone for the evolution of the healthcare industry.
- Predictive Analytics for Healthcare Decision Making: Predictive analytics healthcare solutions are increasingly being used by healthcare leaders to make informed decisions regarding patient care, resource management, and operational improvements. By analyzing historical data, predictive models can help decision-makers anticipate future trends, allocate resources more effectively, and plan interventions that improve both patient outcomes and financial performance.
- Predictive Analytics for Healthcare Providers: Healthcare providers rely on predictive analytics for healthcare decision-making to improve clinical workflows and enhance patient care. For example, hospitals are using predictive tools to reduce readmission rates by identifying patients at risk of complications and providing them with tailored post-discharge care.
- Healthcare Predictive Analytics Use Cases: The use cases of healthcare predictive analytics are diverse and impactful. From predicting disease outbreaks to identifying patients at risk of developing sepsis, predictive healthcare tools are transforming healthcare delivery. One example is the use of predictive analytics for patient outcomes, where hospitals leverage data to predict patient recovery times, enabling them to allocate resources efficiently and provide personalized care.
Why Predictive Analytics is the Future of Personalized Healthcare
As the healthcare industry continues to shift toward personalized care, predictive analytics for healthcare providers is playing a pivotal role in this transition. By enabling a data-driven approach to patient care, healthcare data analytics empowers providers to move beyond reactive treatment and focus on prevention and proactive management.
Predictive analytics healthcare solutions allow healthcare professionals to harness the power of data to make more informed decisions, improve patient outcomes, and reduce healthcare costs. As predictive models in healthcare become more sophisticated, the potential to deliver highly personalized, effective care is boundless.
In conclusion, the integration of predictive analytics for healthcare decision-making will not only reshape how care is delivered but also drive a more efficient and effective healthcare system. From improving chronic disease management to enhancing hospital operations, the future of personalized healthcare lies in the hands of predictive analytics healthcare solutions. By embracing this technology, healthcare providers can unlock new opportunities to deliver better care, reduce costs, and ultimately, improve the lives of patients.
Unlock the power of predictive analytics with Quantzigs expertise in healthcare predictive analytics solutions. Let us help you transform your approach to personalized healthcare.
Start your Free TrialHow Quantzig Can Help with Predictive Analytics in Healthcare
Quantzig is a leading provider of predictive analytics healthcare solutions, offering a suite of advanced healthcare predictive analytics services tailored to meet the evolving needs of healthcare providers, hospitals, and other stakeholders. With our expertise in healthcare data analytics and predictive healthcare tools, we empower organizations to harness the full potential of their data, enabling smarter decision-making and enhanced patient care.
Heres how Quantzig can help:
- Customized Predictive Models in Healthcare
We develop predictive models in healthcare that are specifically designed to address the unique challenges of your organization. Our solutions can forecast patient outcomes, optimize treatment plans, and manage chronic diseases, helping you deliver personalized care thats both efficient and cost-effective. - Comprehensive Data Integration
Quantzig integrates both structured and unstructured data from diverse sources, including EHRs, diagnostic tools, and wearables. Our approach ensures that your predictive analytics for healthcare decision-making is based on the most comprehensive and up-to-date information available, enabling you to make more accurate predictions and improve patient outcomes. - Advanced Predictive Analytics for Patient Outcomes
By leveraging our cutting-edge healthcare analytics software, we enable healthcare providers to predict patient recovery times, anticipate complications, and adjust treatments proactively. Our solutions are tailored to improve operational efficiency and resource allocation, ensuring better care at reduced costs. - Enhancing Chronic Disease Management
Quantzig offers predictive tools that enhance the management of chronic diseases, such as diabetes and heart disease. With our predictive analytics for chronic disease management, you can anticipate disease progression and intervene early, improving patient health while reducing the burden on your healthcare system. - End-to-End Support for Healthcare Providers
We offer a full range of services, from data strategy consulting to solution implementation. Our team of experts works closely with your organization to build and implement robust predictive healthcare analytics frameworks that align with your goals, whether its improving patient care, reducing hospital readmissions, or enhancing operational efficiency.
With Quantzig’s predictive analytics healthcare solutions, healthcare organizations can make more informed decisions, optimize patient outcomes, and drive the shift towards personalized care. Partner with us to unlock the future of healthcare through data-driven innovation.
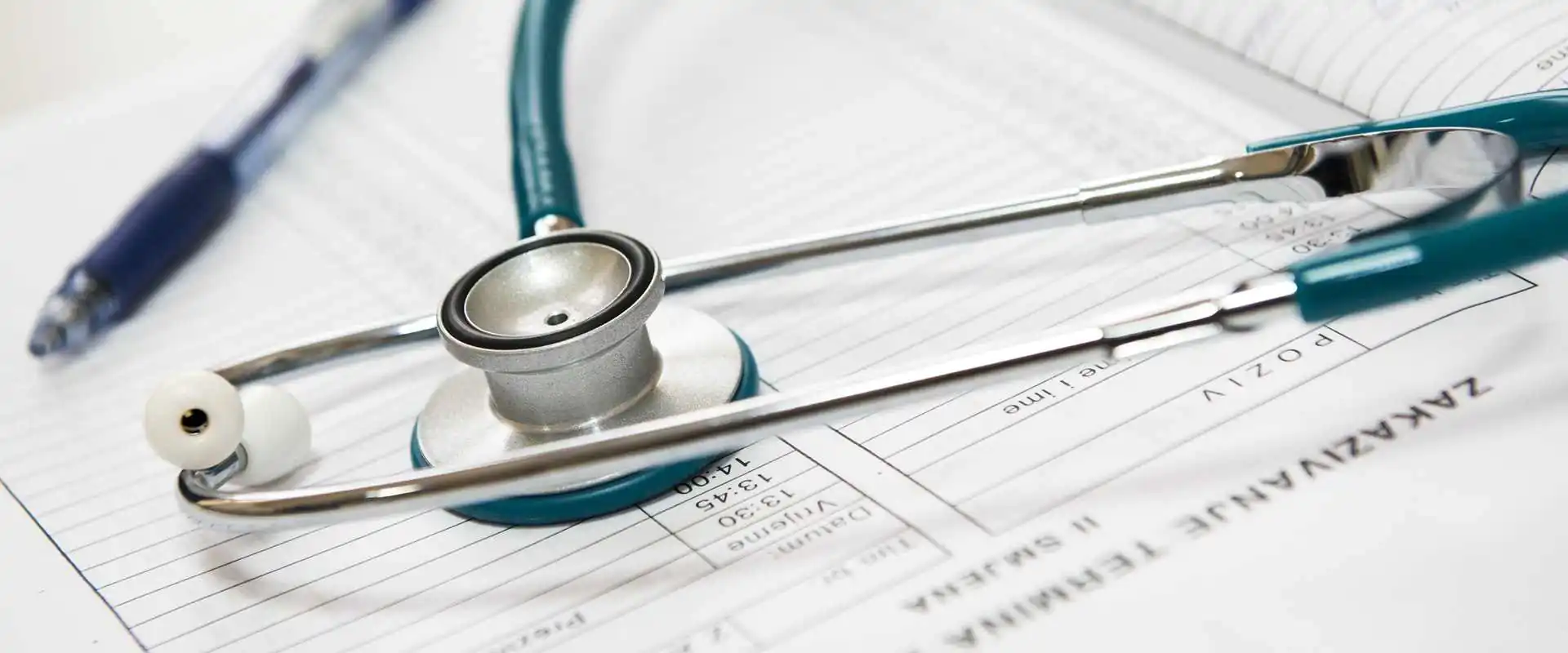
Ready to transform your healthcare delivery? Connect with Quantzig today to discover how our predictive analytics expertise can enhance your patient care and operational efficiency.
Request a Free PilotConnect with us