Written By: Sudeshna Ghosh
Introduction to Response Modeling
Data analytics techniques have undergone dramatic advancements in recent years. This has significantly increased the value of advanced analytics techniques as strategic tools for businesses to achieve desired results. Therefore, companies across industries have started harnessing the power of advanced data analytics techniques to devise strong marketing strategies and boost profitability. The response modeling approach is one such technique that has helped companies to use the information they have mined over the past decade to make data-driven decisions. Today enterprises leveraging this predictive marketing technology have a wealth of unique information, that in turn, is helping them to become “customer-centric” organizations and gain competitive advantage. Response modeling has the potential to help businesses tap into their customer data and offer useful insights for strategic and tactical marketing decisions.
Book a demo to experience the meaningful insights we derive from data through our analytical tools and platform capabilities. Schedule a demo today!
Request a Free DemoWhat is Response Modeling?
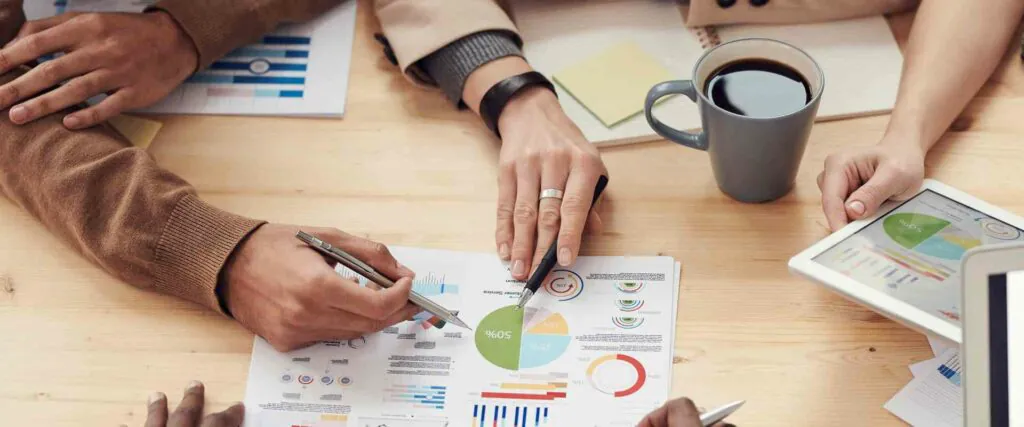
Response modeling is a sophisticated analytical approach that enables organizations to predict and optimize customer responses to marketing campaigns and initiatives. At its core, response modeling involves developing statistical models that correlate customer attributes and behaviors with the likelihood of a desired response, such as making a purchase or engaging with a product. By leveraging advanced algorithms and machine learning, response modeling allows businesses to identify the most promising targets for their marketing efforts, ultimately driving higher returns on investment and more efficient resource allocation.
Whether you’re looking to deepen existing customer relationships or acquire new ones, response modeling provides the insights and predictive capabilities necessary to make data-driven decisions and stay ahead of the competition.
What are the Types of Response Models?
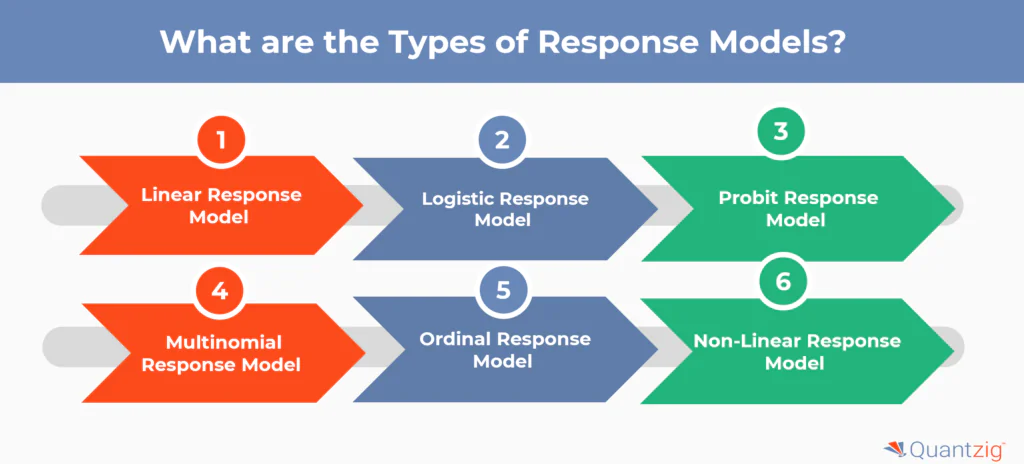
Response models are crucial tools in marketing and sales, enabling businesses to tailor their communication strategies to specific customer needs and preferences. These models help predict and analyze customer responses to various stimuli, such as advertisements, promotions, or product offerings. Here are six key types of response models:
1. Linear Response Model
The linear response model assumes that the response rate is directly proportional to the level of exposure. This means that as the exposure to a stimulus increases, the response rate also increases in a linear fashion. This model is useful for predicting the impact of advertising campaigns or promotional activities on customer engagement.
2. Logistic Response Model
The logistic response model is a more sophisticated version of the linear response model. It takes into account the saturation point, where further increases in exposure do not lead to a proportional increase in response. This model is useful for understanding how customer response rates change over time and how to optimize marketing efforts.
3. Probit Response Model
The probit response model is a statistical technique used to analyze binary response data, where the response is either yes or no. This model is useful for understanding how various factors influence customer decisions, such as purchasing a product or subscribing to a service.
4. Multinomial Response Model
The multinomial response model is used to analyze categorical response data, where the response can take on multiple categories. This model is useful for understanding how customers respond to different product features, pricing strategies, or marketing messages.
5. Ordinal Response Model
The ordinal response model is used to analyze response data that has a natural order or ranking. This model is useful for understanding how customers respond to different levels of service quality, product features, or customer support.
6. Non-Linear Response Model
The non-linear response model is used to analyze response data that does not follow a linear or logistic pattern. This model is useful for understanding how customers respond to complex marketing strategies or product offerings that involve multiple variables.
These response models provide valuable insights into customer behavior and preferences, enabling businesses to refine their marketing strategies and improve customer engagement. By understanding how customers respond to different stimuli, businesses can optimize their marketing efforts and achieve better results.
How Can an Effective Response Modeling Framework Help in Predicting Customer Behavior?
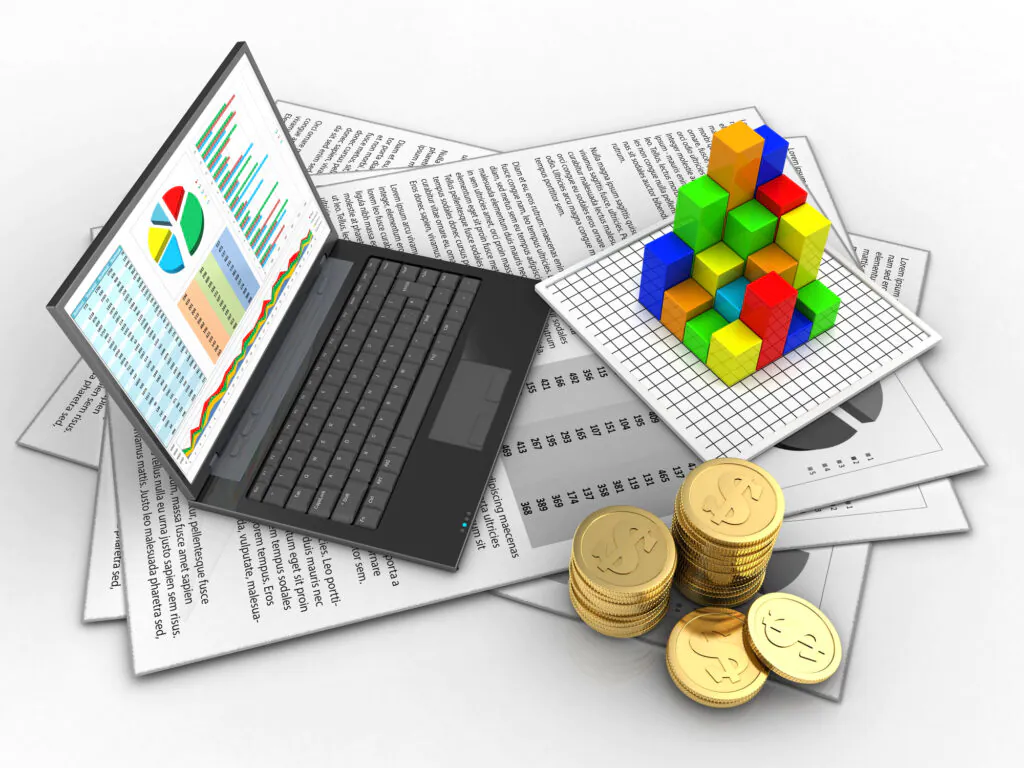
The response modeling approach helps businesses to take into account the interaction of different data elements and identify the people on a list who are most likely to take the desired action. Also, the response modeling approach assists companies in predicting the certain types of customers purchasing behavior and the likelihood of churn. As a result, companies can optimize their marketing strategies such as email send frequency, sales staff time, and discounts.
Using advanced analytics techniques like response modeling, organizations can predict customer buying patterns, prevent fraud, and make crucial business decisions. With insightful statistics and advanced data analysis techniques, companies are starting to develop methodologies to make scientific, data-driven predictions as to what their customers are likely to do in the next buying season. Furthermore, response modeling helps in developing an analytical framework that can help to forecast the impact of a change in market strategy or the launch of a new product or service that is closely related to demographic biases of customers.
Experience the advantages firsthand by testing a customized complimentary pilot designed to address your specific requirements. Pilot studies are non-committal in nature.
Request a Free PilotPropensity Models – Response Models Predictive Analytics
Propensity models are a type of predictive analytics used to forecast customer behavior. These models analyze various factors such as customer interactions, demographics, and transaction history to predict the likelihood of a customer performing a specific action, like making a purchase. By identifying high-propensity customers, businesses can optimize marketing efforts, allocate resources effectively, and increase conversions.
Propensity models are particularly useful for businesses looking to personalize their marketing strategies and target high-value customers. By analyzing customer data, these models can identify patterns and trends that indicate a customer’s likelihood of responding to a particular offer or promotion. This information can be used to tailor marketing campaigns, improve customer engagement, and drive revenue growth.
Propensity models can be applied to a wide range of business scenarios, including customer acquisition, retention, and upselling. By leveraging these models, businesses can make data-driven decisions and optimize their marketing strategies to achieve better results.
Why Choose Quantzig?
Quantzig’s response modeling capabilities help companies to develop proactive targeting frameworks to understand customer responses to individual tactics, an order of exposure, and both combined. At Quantzig, we understand the difference a robust response modelling approach can bring to your business. Our solutions help you identify the right tactic, order, and frequency to target the customers to maximize returns. By leveraging analytics solutions, businesses can gain end to end visibility into their customer’s journeys and identify the right opportunities to boost sales.
Get started with your complimentary trial today and delve into our platform without any obligations. Explore our wide range of customized, consumption driven analytical solutions services built across the analytical maturity levels.
Start your Free Trial todayConclusion
Response modeling techniques are transforming marketing by leveraging predictive analytics for effective customer acquisition and customer retention. Using propensity models, businesses can predict the likelihood to buy, optimizing resource allocation. Incremental response modeling identifies the additional impact of marketing activities, refining these efforts.
For multichannel retailers of sports goods and B2B marketers, predictive personalization software (PPS) customizes outreach based on customer behaviors, enhancing engagement and sales. Response models aid in lead scoring, prioritizing prospects by their predicted responsiveness, driving more targeted and efficient predictive marketing campaigns.
By predicting the likelihood of buying, businesses can fine-tune strategies, boosting conversion rates and customer retention. Integrating predictive insight into marketing not only improves campaign efficiency but also enriches customer experience. Embracing these advanced response modeling techniques is paving the way for a more predictive and personalized marketing landscape.